· 8 min read
Predictive analytics set to become more valuable in light of rising CPIs
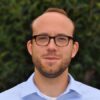
Julian Runge
Head of Analytics & Data Science at Wooga.
Say you produce and market mobile apps for iPhones. You have 1 million active users in the US. Now what are these users economically worth to you? Let’s do a little thought experiment: Say you were to sell them all at market cost-per-install (CPI) to other mobile developers. In January 2012 that would have yielded 1.3 million USD – very roughly speaking and abstracting from transaction cost and economies of scale. The same thought experiment would have generated around 2 million USD in December 2012. And by now, it would generate a staggering 3.4 million USD for you [1]. That’s quite a change, isn’t it?
The industry is consolidating. A few main players generate big revenues and spend big on user acquisition. The market is crowded with pretty good apps, marginalizing viral and organic growth. For developers with thin capital layers it becomes very difficult to acquire users. It is always a worthwhile effort to retain players. I’m not talking about development iterations to increase retention, but about the customer relationship management (CRM) layer that you can put on top of your games. This sounds very much like classical marketing jargon (which it is). For mobile apps this entails customer support, community management and many of the more traditional approaches. However, the transparency of the mobile user through tracking – at least within a company’s own products – enables a plethora of analytical methods to be applied.
Let’s call these quantitative CRM. Take the examples of cross linking (from a sending to a receiving game inside your portfolio) and incentivizing (e.g. giving out gifts). You can do that by just showing banners/giving gifts to every player or maybe based on some simple heuristic, e.g. target all players in level 30 plus. However, if you incentivize and crosslink too much and too early, you may reduce both your players’ expected lifetime and spending in a game. This is what the upper part in figure 1 shows. Player 1 has the longest lifetime and spends money in the game. Player 2 and 3 are non-converting players with different lifetimes. While you crosslink player 3 at the optimal point in time, you potentially harm your user numbers (example of player 2) and revenue (player 1) in the sending game if your crosslinking effort is successful. Hence, what you want to know is when your users are going to leave your sending game anyways. Then, sending them to another game or giving them some free stuff comes at no cost to you.
The lower part of figure 1 shows this case: A perfect prediction model enables you to crosslink each player at the optimal point – the end of her product lifetime.
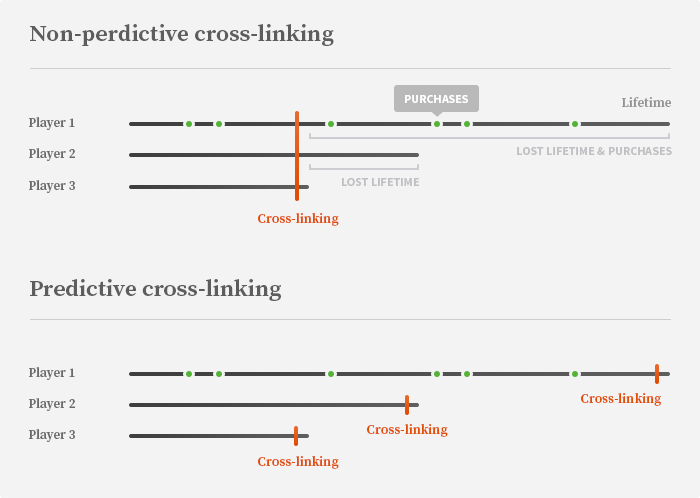
That’s why we sat down at Wooga and looked into what analytics can do for us there. We wanted to know if we can predict player lifetime and if there is value in quantitative CRM. We came across some inspiring articles on the web and decided to try something similar for our games.
Learnings
During the process, we picked up some precious insights that we want to share before we get into the nitty gritty of how we got there.
- As Dmitry Nozhnin also finds in his Gamasutra articles, individual activity and engagement metrics carry the greatest predictive ability.
- The behavioral patterns of players are less pronounced for highly casual games that require less commitment and less constant engagement of players. This makes it more difficult to predict churn for this type of games.
- Similarly, churn prediction is easier for more engaged segments of a game’s user base. So, running the prediction on a highly engaged segment can be a good idea.
- Contacting users before instead of after the churn event is bound to increase the effectiveness of a communication effort multiple times.
- Incentives such as free gifts cannot retain players when they reach the end of their lifetime; cross-linking to other games (in your portfolio) seems a more promising way to go. Or changing the gameplay experience way ahead of the churn event through e.g. adaptive gameplay.
Methodology
We especially want to talk about two of Wooga’s most successful games here, Monster World and Diamond Dash. Diamond Dash is a highly casual arcade game like Tetris or Bejeweled where players have to clear a board of colored diamonds as quickly as possible in a specified time in order to crack the high score. Monster World represents the other end of the spectrum of casual games – a more deeply engaging casual game. It is a farming game with a twist which simulates the economics of running a farm. Compared to Diamond Dash, Monster World requires more commitment and constant engagement in order to keep the garden running. Both games have abundant historical tracking data available and the games are at a mature stage where no drastic game feature changes are likely to occur.
We formulated the churn prediction as a binary classification problem where the prediction model is trained using historical tracking data. Given new tracking data the model will be able to output a binary variable indicating whether a player is about to churn or not. After a series of data cleansing and data transformation procedures, we first constructed two high quality and well-behaved datasets for the two games under study and carefully selected the features to use for the prediction model. Empirical test results showed that most recent general activity data such as a time series of number of logins for the last 14 days together with some other player profile data (such as days passed since the player first played the game) carry the most predictive power. If you are interested in details on this, please take a look at these papers: Churn Prediction for High-Value Players in Casual Social Games and Predicting Player Churn in the Wild, these are highly informative on this regard. For the more applied audience, let’s move to the use case.’
Use case
We decided to try out incentivization for high-value players in Monster World. We implemented an A/B test where each group received a different churn management policy. In the first group called the heuristic group, we applied what every company with a simple tracking setup can do: Send incentives to churned (i.e. inactive for 14 consecutive days) high-value players. In the second group called the predictive group, we sent incentives to all churned high-value players and all players whom the model predicted to churn within the next week. Finally, we kept a control group where we did not apply any churn management.
The A/B test ran for a month. During this time we sent incentivized links through Facebook notifications and e-mails. When a player clicked the link, she got a sizeable package of in-game currency, worth approximately 10 USD. The idea being that this injection would trigger the player to keep playing. Unfortunately, the achieved improvements in churn rate and revenue in both the predictive and heuristic group were not statistically significant when compared to the control group. Also the difference between the predictive and heuristic group remained insignificant. Our incentives hence did not succeed in retaining churning high-value players and the prediction model could not change that.
This was the bad news, here comes the good: When looking at the click-through rates of the e-mails and Facebook notifications we sent out, we saw a huge difference between the predictive and heuristic churn management approaches. Figure 3 and 4 illustrate the e-mail campaign and Facebook notifications funnel for the two test groups. The share of claimed gift links is much higher for the predictive group. 18% of the Facebook notifications were converted in the predictive group, while this number is only 4% in the heuristic group. For e-mail, the numbers are 11% and 2% respectively.
Figure 2. Email campaign funnel
Figure 3. Facebook notifications funnel
Business impact
So, even if the business impact of incentivization appears to be minor, churn prediction and quantitative CRM hold huge potential. For engaging casual games churn prediction works. And it can be used to crosslink players, namely high-value players, before the actual churn event. That’s where your product strategy kicks in. The most straightforward thing to do is to send players to a game from the same genre in your own portfolio. This is predestinated to save you a whole lot in user acquisition spending. Every player successfully crosslinked saves you one CPI. The higher the CPI, the more you save.
For Supercell’s Boom Beach rumors already have it that it is there to catch up players who are tired of Clash of Clans. It will be interesting to see if crosslinking to the same genre or different genres or even giving players a choice works best. Along these lines Sifa et al. started looking into recommender systems for games. I am convinced that we will see strategic product portfolio management more and more in the days to come. And with it the rise of quantitative CRM.